Is In-Context Learning in Large Language Models Bayesian? A Martingale Perspective
Best AI papers explained - Een podcast door Enoch H. Kang
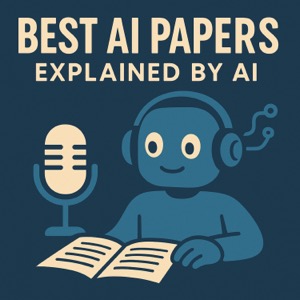
Categorieën:
This academic paper explores whether the in-context learning (ICL) process in Large Language Models (LLMs) behaves like Bayesian inference. The authors use the martingale property, a key characteristic of Bayesian systems with exchangeable data, as a framework for their analysis. They demonstrate that violations of this property and deviations in how LLMs' uncertainty scales with more data provide evidence that ICL is not Bayesian. The findings suggest that LLMs lack a principled understanding of uncertainty in exchangeable scenarios, raising concerns about their reliability in applications where data order doesn't matter or where quantifiable uncertainty is critical.