Test-Time Alignment Via Hypothesis Reweighting
Best AI papers explained - Een podcast door Enoch H. Kang
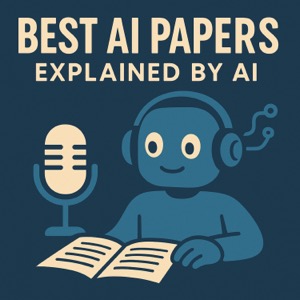
Categorieën:
This paper presents HYRE, a method for quickly adapting large pretrained models to underspecified tasks like personalization or handling distribution shifts. It works by first training a single neural network that represents an ensemble of diverse models. At test time, using a small set of labeled examples from the target distribution, HYRE dynamically reweights the ensemble members based on their performance, selecting the combination of models best suited for the specific task without retraining. Experiments demonstrate that HYRE outperforms prior methods and fine-tuning in low-data regimes, achieving strong results with minimal computational overhead and a few adaptation samples. The authors show that diverse ensembles trained with random priors can capture distinct functional variations, enabling effective adaptation to individual preferences and other underspecified scenarios.