The Alternative Annotator Test for LLM-as-a-Judge: How to Statistically Justify Replacing Human Annotators with LLMs
Best AI papers explained - Een podcast door Enoch H. Kang
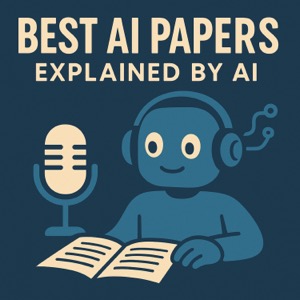
Categorieën:
This paper proposes the Alternative Annotator Test (alt-test), a novel statistical method for determining if a Large Language Model (LLM) can reliably substitute for human annotators in research tasks across various fields. The test involves comparing LLM annotations to those of a small group of human annotators on a subset of data to see if the LLM aligns better with the group than individual humans do. It also introduces the Average Advantage Probability, a measure for comparing the performance of different LLM judges. Experiments conducted on diverse datasets and with different LLMs demonstrate that some LLMs can pass the alt-test, particularly closed-source models and those utilizing few-shot prompting, suggesting their potential as alternative annotators in certain scenarios while highlighting the need for rigorous evaluation.