Charting the Path of Riskified's Data Platform Journey
Data Engineering Podcast - Een podcast door Tobias Macey - Zondagen
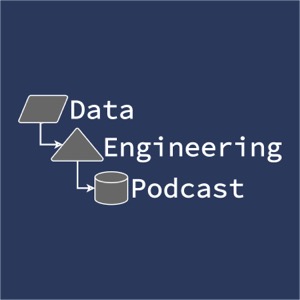
Categorieën:
Summary Building a data platform is a journey, not a destination. Beyond the work of assembling a set of technologies and building integrations across them, there is also the work of growing and organizing a team that can support and benefit from that platform. In this episode Inbar Yogev and Lior Winner share the journey that they and their teams at Riskified have been on for their data platform. They also discuss how they have established a guild system for training and supporting data professionals in the organization. Announcements Hello and welcome to the Data Engineering Podcast, the show about modern data management When you’re ready to build your next pipeline, or want to test out the projects you hear about on the show, you’ll need somewhere to deploy it, so check out our friends at Linode. With their new managed database service you can launch a production ready MySQL, Postgres, or MongoDB cluster in minutes, with automated backups, 40 Gbps connections from your application hosts, and high throughput SSDs. Go to dataengineeringpodcast.com/linode today and get a $100 credit to launch a database, create a Kubernetes cluster, or take advantage of all of their other services. And don’t forget to thank them for their continued support of this show! Atlan is the metadata hub for your data ecosystem. Instead of locking your metadata into a new silo, unleash its transformative potential with Atlan’s active metadata capabilities. Push information about data freshness and quality to your business intelligence, automatically scale up and down your warehouse based on usage patterns, and let the bots answer those questions in Slack so that the humans can focus on delivering real value. Go to dataengineeringpodcast.com/atlan today to learn more about how Atlan’s active metadata platform is helping pioneering data teams like Postman, Plaid, WeWork & Unilever achieve extraordinary things with metadata and escape the chaos. Modern data teams are dealing with a lot of complexity in their data pipelines and analytical code. Monitoring data quality, tracing incidents, and testing changes can be daunting and often takes hours to days or even weeks. By the time errors have made their way into production, it’s often too late and damage is done. Datafold built automated regression testing to help data and analytics engineers deal with data quality in their pull requests. Datafold shows how a change in SQL code affects your data, both on a statistical level and down to individual rows and values before it gets merged to production. No more shipping and praying, you can now know exactly what will change in your database! Datafold integrates with all major data warehouses as well as frameworks such as Airflow & dbt and seamlessly plugs into CI workflows. Visit dataengineeringpodcast.com/datafold today to book a demo with Datafold. Tired of deploying bad data? Need to automate data pipelines with less red tape? Shipyard is the premier data orchestration platform built to help your data team quickly launch, monitor, and share workflows in a matter of minutes. Build powerful workflows that connect your entire data stack end-to-end with a mix of your code and their open-source, low-code templates. Once launched, Shipyard makes data observability easy with logging, alerting, and retries that will catch errors before your business team does. So whether you’re ingesting data from an API, transforming it with dbt, updating BI tools, or sending data alerts, Shipyard centralizes these operations and handles the heavy lifting so your data team can finally focus on what they’re good at — solving problems with data. Go to dataengineeringpodcast.com/shipyard to get started automating with their free developer plan today! Your host is Tobias Macey and today I’m interviewing Inbar Yogev and Lior Winner about the data platform that the team at Riskified are building to power their fraud management service Interview Introduction How did you get involved in the area of data management? What does Riskified do? Can you describe the role of data at Riskified? What are some of the core types and sources of information that you are dealing with? Who/what are the primary consumers of the data that you are responsible for? What are the team structures that you have tested for your data professionals? What is the composition of your data roles? (e.g. ML engineers, data engineers, data scientists, data product managers, etc.) What are the organizational constraints that have the biggest impact on the design and usage of your data systems? Can you describe the current architecture of your data platform? What are some of the most notable evolutions/redesigns that you have gone through? What is your process for establishing and evaluating selection criteria for any new technologies that you adopt? How do you facilitate knowledge sharing between data professionals? What have you found to be the most challenging technological and organizational complexities that you have had to address on the path to your current state? What are the methods that you use for staying up to date with the data ecosystem? (opportunity to discuss Haya Data conference) In your role as organizers of the Haya Data conference, what are some of the insights that you have gained into the present state and future trajectory of the data community? What are the most interesting, innovative, or unexpected ways that you have seen the Riskified data platform used? What are the most interesting, unexpected, or challenging lessons that you have learned while working on the data platform for Riskified? What do you have planned for the future of your data platform? Contact Info Inbar LinkedIn Lior LinkedIn Parting Question From your perspective, what is the biggest gap in the tooling or technology for data management today? Closing Announcements Thank you for listening! Don’t forget to check out our other shows. Podcast.__init__ covers the Python language, its community, and the innovative ways it is being used. The Machine Learning Podcast helps you go from idea to production with machine learning. Visit the site to subscribe to the show, sign up for the mailing list, and read the show notes. If you’ve learned something or tried out a project from the show then tell us about it! Email [email protected]) with your story. To help other people find the show please leave a review on Apple Podcasts and tell your friends and co-workers Links Riskified ADABAS Aerospike Podcast Episode Neo4J Kafka Delta Lake Podcast Episode Databricks Snowflake Podcast Episode Tableau Looker Podcast Episode Redshift Event Sourcing Avro hayaData Conference Data Mesh Data Catalog Data Governance MLOps Podcast Episode The intro and outro music is from The Hug by The Freak Fandango Orchestra / CC BY-SA Support Data Engineering Podcast