Data Exploration For Business Users Powered By Analytics Engineering With Lightdash
Data Engineering Podcast - Een podcast door Tobias Macey - Zondagen
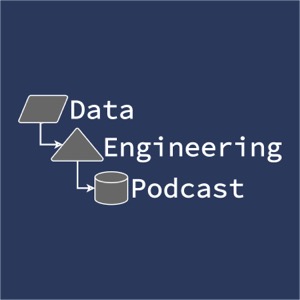
Categorieën:
Summary The market for business intelligence has been going through an evolutionary shift in recent years. One of the driving forces for that change has been the rise of analytics engineering powered by dbt. Lightdash has fully embraced that shift by building an entire open source business intelligence framework that is powered by dbt models. In this episode Oliver Laslett describes why dashboards aren’t sufficient for business analytics, how Lightdash promotes the work that you are already doing in your data warehouse modeling with dbt, and how they are focusing on bridging the divide between data teams and business teams and the requirements that they have for data workflows. Announcements Hello and welcome to the Data Engineering Podcast, the show about modern data management When you’re ready to build your next pipeline, or want to test out the projects you hear about on the show, you’ll need somewhere to deploy it, so check out our friends at Linode. With their managed Kubernetes platform it’s now even easier to deploy and scale your workflows, or try out the latest Helm charts from tools like Pulsar and Pachyderm. With simple pricing, fast networking, object storage, and worldwide data centers, you’ve got everything you need to run a bulletproof data platform. Go to dataengineeringpodcast.com/linode today and get a $100 credit to try out a Kubernetes cluster of your own. And don’t forget to thank them for their continued support of this show! Are you bored with writing scripts to move data into SaaS tools like Salesforce, Marketo, or Facebook Ads? Hightouch is the easiest way to sync data into the platforms that your business teams rely on. The data you’re looking for is already in your data warehouse and BI tools. Connect your warehouse to Hightouch, paste a SQL query, and use their visual mapper to specify how data should appear in your SaaS systems. No more scripts, just SQL. Supercharge your business teams with customer data using Hightouch for Reverse ETL today. Get started for free at dataengineeringpodcast.com/hightouch. Modern Data teams are dealing with a lot of complexity in their data pipelines and analytical code. Monitoring data quality, tracing incidents, and testing changes can be daunting and often takes hours to days. Datafold helps Data teams gain visibility and confidence in the quality of their analytical data through data profiling, column-level lineage and intelligent anomaly detection. Datafold also helps automate regression testing of ETL code with its Data Diff feature that instantly shows how a change in ETL or BI code affects the produced data, both on a statistical level and down to individual rows and values. Datafold integrates with all major data warehouses as well as frameworks such as Airflow & dbt and seamlessly plugs into CI workflows. Go to dataengineeringpodcast.com/datafold today to start a 30-day trial of Datafold. Your host is Tobias Macey and today I’m interviewing Oliver Laslett about Lightdash, an open source business intelligence system powered by your dbt models Interview Introduction How did you get involved in the area of data management? Can you describe what Lightdash is and the story behind it? What are the main goals of the project? Who are the target users, and how has that profile informed your feature priorities? Business intelligence is a market that has gone through several generational shifts, with products targeting numerous personas and purposes. What are the capabilities that make Lightdash stand out from the other options? Can you describe how Lightdash is architected? How have the design and goals of the system changed or evolved since you first began working on it? What have been the most challenging engineering problems that you have dealt with? How does the approach that you are taking with Lightdash compare to systems such as Transform and Metriql that aim to provide a dedicated metrics layer? Can you describe the workflow for someone building an analysis in Lightdash? What are the points of collaboration around Lightdash for different roles in the organization? What are the methods that you use to expose information about the state of the underlying dbt models to the end users? How do they use that information in their exploration and decision making? What was your motivation for releasing Lightdash as open source? How are you handling the governance and long-term viability of the project? What are the most interesting, innovative, or unexpected ways that you have seen Lightdash used? What are the most interesting, unexpected, or challenging lessons that you have learned while working on Lightdash? When is Lightdash the wrong choice? What do you have planned for the future of Lightdash? Contact Info LinkedIn owlas on GitHub Parting Question From your perspective, what is the biggest gap in the tooling or technology for data management today? Links Lightdash Looker Podcast Episode PowerBI Podcast Episode Redash Podcast Episode Metabase Podcast Episode dbt Podcast Episode Superset Podcast Episode Streamlit Podcast Episode Kubernetes JDBC SQLAlchemy SQLPad Singer Podcast Episode Airbyte Podcast Episode Meltano Podcast Episode Transform Podcast Episode Metriql Podcast Episode Cube.js OpenLineage Podcast Episode dbt Packages Rudderstack PostHog Podcast Interview Firebolt Podcast Interview The intro and outro music is from The Hug by The Freak Fandango Orchestra / CC BY-SA Support Data Engineering Podcast