Data Quality Starts At The Source
Data Engineering Podcast - Een podcast door Tobias Macey - Zondagen
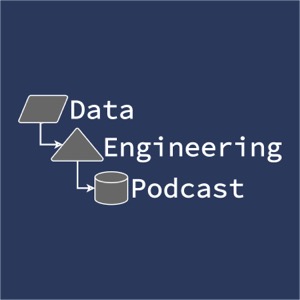
Categorieën:
Summary The most important gauge of success for a data platform is the level of trust in the accuracy of the information that it provides. In order to build and maintain that trust it is necessary to invest in defining, monitoring, and enforcing data quality metrics. In this episode Michael Harper advocates for proactive data quality and starting with the source, rather than being reactive and having to work backwards from when a problem is found. Announcements Hello and welcome to the Data Engineering Podcast, the show about modern data management When you’re ready to build your next pipeline, or want to test out the projects you hear about on the show, you’ll need somewhere to deploy it, so check out our friends at Linode. With their managed Kubernetes platform it’s now even easier to deploy and scale your workflows, or try out the latest Helm charts from tools like Pulsar and Pachyderm. With simple pricing, fast networking, object storage, and worldwide data centers, you’ve got everything you need to run a bulletproof data platform. Go to dataengineeringpodcast.com/linode today and get a $100 credit to try out a Kubernetes cluster of your own. And don’t forget to thank them for their continued support of this show! Atlan is a collaborative workspace for data-driven teams, like Github for engineering or Figma for design teams. By acting as a virtual hub for data assets ranging from tables and dashboards to SQL snippets & code, Atlan enables teams to create a single source of truth for all their data assets, and collaborate across the modern data stack through deep integrations with tools like Snowflake, Slack, Looker and more. Go to dataengineeringpodcast.com/atlan today and sign up for a free trial. If you’re a data engineering podcast listener, you get credits worth $3000 on an annual subscription Modern Data teams are dealing with a lot of complexity in their data pipelines and analytical code. Monitoring data quality, tracing incidents, and testing changes can be daunting and often takes hours to days. Datafold helps Data teams gain visibility and confidence in the quality of their analytical data through data profiling, column-level lineage and intelligent anomaly detection. Datafold also helps automate regression testing of ETL code with its Data Diff feature that instantly shows how a change in ETL or BI code affects the produced data, both on a statistical level and down to individual rows and values. Datafold integrates with all major data warehouses as well as frameworks such as Airflow & dbt and seamlessly plugs into CI workflows. Go to dataengineeringpodcast.com/datafold today to start a 30-day trial of Datafold. Your host is Tobias Macey and today I’m interviewing Michael Harper about definitions of data quality and where to define and enforce it in the data platform Interview Introduction How did you get involved in the area of data management? What is your definition for the term "data quality" and what are the implied goals that it embodies? What are some ways that different stakeholders and participants in the data lifecycle might disagree about the definitions and manifestations of data quality? The market for "data quality tools" has been growing and gaining attention recently. How would you categorize the different approaches taken by open source and commercial options in the ecosystem? What are the tradeoffs that you see in each approach? (e.g. data warehouse as a chokepoint vs quality checks on extract) What are the difficulties that engineers and stakeholders encounter when identifying and defining information that is necessary to identify issues in their workflows? Can you describe some examples of adding data quality checks to the beginning stages of a data workflow and the kinds of issues that can be identified? What are some ways that quality and observability metrics can be aggregated across multiple pipeline stages to identify more complex issues? In application observability the metrics across multiple processes are often associated with a given service. What is the equivalent concept in data platform observabiliity? In your work at Databand what are some of the ways that your ideas and assumptions around data quality have been challenged or changed? What are the most interesting, innovative, or unexpected ways that you have seen Databand used? What are the most interesting, unexpected, or challenging lessons that you have learned while working at Databand? When is Databand the wrong choice? What do you have planned for the future of Databand? Contact Info LinkedIn Parting Question From your perspective, what is the biggest gap in the tooling or technology for data management today? Links Databand Clean Architecture (affiliate link) Great Expectations Deequ The intro and outro music is from The Hug by The Freak Fandango Orchestra / CC BY-SA Support Data Engineering Podcast