What Does It Really Mean To Do MLOps And What Is The Data Engineer's Role?
Data Engineering Podcast - Een podcast door Tobias Macey - Zondagen
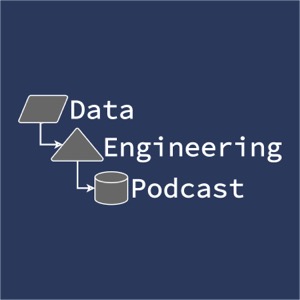
Categorieën:
Summary Putting machine learning models into production and keeping them there requires investing in well-managed systems to manage the full lifecycle of data cleaning, training, deployment and monitoring. This requires a repeatable and evolvable set of processes to keep it functional. The term MLOps has been coined to encapsulate all of these principles and the broader data community is working to establish a set of best practices and useful guidelines for streamlining adoption. In this episode Demetrios Brinkmann and David Aponte share their perspectives on this rapidly changing space and what they have learned from their work building the MLOps community through blog posts, podcasts, and discussion forums. Announcements Hello and welcome to the Data Engineering Podcast, the show about modern data management When you’re ready to build your next pipeline, or want to test out the projects you hear about on the show, you’ll need somewhere to deploy it, so check out our friends at Linode. With their managed Kubernetes platform it’s now even easier to deploy and scale your workflows, or try out the latest Helm charts from tools like Pulsar and Pachyderm. With simple pricing, fast networking, object storage, and worldwide data centers, you’ve got everything you need to run a bulletproof data platform. Go to dataengineeringpodcast.com/linode today and get a $100 credit to try out a Kubernetes cluster of your own. And don’t forget to thank them for their continued support of this show! This episode is brought to you by Acryl Data, the company behind DataHub, the leading developer-friendly data catalog for the modern data stack. Open Source DataHub is running in production at several companies like Peloton, Optum, Udemy, Zynga and others. Acryl Data provides DataHub as an easy to consume SaaS product which has been adopted by several companies. Signup for the SaaS product at dataengineeringpodcast.com/acryl RudderStack helps you build a customer data platform on your warehouse or data lake. Instead of trapping data in a black box, they enable you to easily collect customer data from the entire stack and build an identity graph on your warehouse, giving you full visibility and control. Their SDKs make event streaming from any app or website easy, and their state-of-the-art reverse ETL pipelines enable you to send enriched data to any cloud tool. Sign up free… or just get the free t-shirt for being a listener of the Data Engineering Podcast at dataengineeringpodcast.com/rudder. Your host is Tobias Macey and today I’m interviewing Demetrios Brinkmann and David Aponte about what you need to know about MLOps as a data engineer Interview Introduction How did you get involved in the area of data management? Can you describe what MLOps is? How does it relate to DataOps? DevOps? (is it just another buzzword?) What is your interest and involvement in the space of MLOps? What are the open and active questions in the MLOps community? Who is responsible for MLOps in an organization? What is the role of the data engineer in that process? What are the core capabilities that are necessary to support an "MLOps" workflow? How do the current platform technologies support the adoption of MLOps workflows? What are the areas that are currently underdeveloped/underserved? Can you describe the technical and organizational design/architecture decisions that need to be made when endeavoring to adopt MLOps practices? What are some of the common requirements for supporting ML workflows? What are some of the ways that requirements become bespoke to a given organization or project? What are the opportunities for standardization or consolidation in the tooling for MLOps? What are the pieces that are always going to require custom engineering? What are the most interesting, innovative, or unexpected approaches to MLOps workflows/platforms that you have seen? What are the most interesting, unexpected, or challenging lessons that you have learned while working on supporting the MLOps community? What are your predictions for the future of MLOps? What are you keeping a close eye on? Contact Info Demetrios LinkedIn @Dpbrinkm on Twitter Medium David LinkedIn @aponteanalytics on Twitter aponte411 on GitHub Parting Question From your perspective, what is the biggest gap in the tooling or technology for data management today? Links MLOps Community Everybody Lies: Big Data, New Data, and What the Internet Can Tell Us About Who We Really Are by Seth Stephens-Davidowitz (affiliate link) MLOps DataOps DevOps The Sequence Newsletter Neptune.ai Algorithmia Kubeflow The intro and outro music is from The Hug by The Freak Fandango Orchestra / CC BY-SA Support Data Engineering Podcast