074 - Why a Former Microsoft ML/AI Researcher Turned to Design to Create Intelligent Products from Messy Data with Abhay Agarwal, Founder of Polytopal
Experiencing Data w/ Brian T. O’Neill (UX for AI Data Products, SAAS Analytics, Data Product Management) - Een podcast door Brian T. O’Neill from Designing for Analytics - Dinsdagen
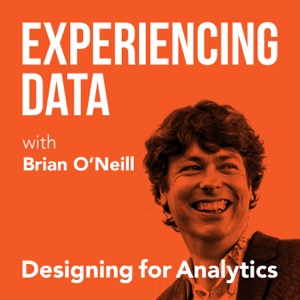
Categorieën:
Episode Description The challenges of design and AI are exciting ones to face. The key to being successful in that space lies in many places, but one of the most important is instituting the right design language. For Abhay Agarwal, Founder of Polytopal, when he began to think about design during his time at Microsoft working on systems to help the visually impared, he realized the necessity of a design language for AI. Stepping away from that experience, he leaned into how to create a new methodology of design centered around human needs. His efforts have helped shift the lens of design towards how people solve problems. In this episode, Abhay and I go into details on a snippet from his course page for the Stanford d. where he claimed that “the foreseeable future would not be well designed, given the difficulty of collaboration between disciplines.” Abhay breaks down how he thinks his design language for AI should work and how to build it out so that everyone in an organization can come to a more robust understanding of AI. We also discuss the future of designers and AI and the ebb and flow of changing, learning, and moving forward with the AI narrative. In our chat, we covered: Abhay’s background in AI research and what happened to make him move towards design as a method to produce intelligence from messy data. (1:01) Why Abhay has come up with a new design language called Lingua Franca for machine learning products [and his course on this at Stanford’s d.school]. (3:21) How to become more human-centered when building AI products, what ethnographers can uncover, and some of Abhay’s real-world examples. (8:06) Biases in design and the challenges in developing a shared language for both designers and AI engineers. (15:59) Discussing interpretability within black box models using music recommendation systems, like Spotify, as an example. (19:53) How “unlearning” solves one of the biggest challenges teams face when collaborating and engaging with each other. (27:19) How Abhay is shaping the field of design and ML/AI -- and what’s in store for Lingua Franca. (35:45) Quotes from Today's Episode “I certainly don’t think that one needs to hit the books on design thinking or listen to a design thinker describe their process in order to get the fundamentals of a human-centered design process. I personally think it’s something that one can describe to you within the span of a single conversation, and someone who is listening to that can then interpret that and say, ‘Okay well, what am I doing that could be more human-centered?’ In the AI space, I think this is the perennial question.” - Abhay Agarwal (@Denizen_Kane) (6:30) “Show me a company where designers feel at an equivalent level to AI engineers when brainstorming technology? It just doesn’t happen. There’s a future state that I want us to get to that I think is along those lines. And so, I personally see this as, kind of, a community-wide discussion, engagement, and multi-strategy approach.” - Abhay Agarwal (@Denizen_Kane) (18:25) “[Discussing ML data labeling for music recommenders] I was just watching a video about drum and bass production, and they were talking about, “Or you can write your bass lines like this”—and they call it reggaeton. And it’s not really reggaeton at all, which was really born in Puerto Rico. And Brazil does the same thing with their versions of reggae. It’s not the one-drop reggae we think of Bob Marley and Jamaica. So already, we’ve got labeling issues—and they’re not even wrong; it’s just that that’s the way one person might interpret what these musical terms mean” - Brian O’Neill (@rhythmspice) (25:45) “There is a new kind of hybrid role that is emerging that we play into...which is an AI designer, someone who is very proficient with understanding the dynamics of AI systems. The same way that we have digital UX designers, app designers—there had to be apps before they could be app designers—there is now AI, and then there can thus