105 - Defining “Data Product” the Producty Way and the Non-technical Skills ML/AI Product Managers Need
Experiencing Data w/ Brian T. O’Neill (UX for AI Data Products, SAAS Analytics, Data Product Management) - Een podcast door Brian T. O’Neill from Designing for Analytics - Dinsdagen
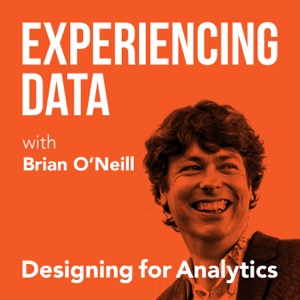
Categorieën:
Today I’m discussing something we’ve been talking about a lot on the podcast recently - the definition of a “data product.” While my definition is still a work in progress, I think it’s worth putting out into the world at this point to get more feedback. In addition to sharing my definition of data products (as defined the “producty way”), on today’s episode definition, I also discuss some of the non-technical skills that data product managers (DPMs) in the ML and AI space need if they want to achieve good user adoption of their solutions. I’ll also share my thoughts on whether data scientists can make good data product managers, what a DPM can do to better understand your users and stakeholders, and how product and UX design factors into this role. Highlights/ Skip to: I introduce my reasons for sharing my definition of a data product (0:46) My definition of data product (7:26) Thinking the “producty” way (8:14) My thoughts on necessary skills for data PMs (in particular, AI & machine learning product management) (12:21) How data scientists can become good data product managers (DPMs) by taking off the data science hat (13:42) Understanding the role of UX design within the context of DPM (16:37) Crafting your sales and marketing strategies to emphasize the value of your product to the people who can use or purchase it (23:07) How to build a team that will help you increase adoption of your data product (30:01) How to build relationships with stakeholders/customers that allow you to find the right solutions for them (33:47) Letting go of a technical identity to develop a new identity as a DPM who can lead a team to build a product that actually gets used (36:32) Quotes from Today’s Episode “This is what’s missing in some of the other definitions that I see around data products [...] they’re not talking about it from the customer of the data product lens. And that orientation sums up all of the work that I’m doing and trying to get you to do as well, which is to put the people at the center of the work that you’re doing and not the data science, engineering, tech, or design. I want you to put the people at the center.” (6:12) “A data product is a data-driven, end-to-end, human-in-the-loop decision support solution that’s so valuable, users would potentially pay to use it.” (7:26) “I want to plunge all the way in and say, ‘if you want to do this kind of work, then you need to be thinking the product-y way.’ And this means inherently letting go of some of the data science-y way of thinking and the data-first kinds of ways of thinking.” (11:46) “I’ve read in a few places that data scientists don’t make for good data product managers. [While it may be true that they’re more introverted,] I don’t think that necessarily means that there’s an inherent problem with data scientists becoming good data product managers. I think the main challenge will be—and this is the same thing for almost any career transitioning into product management—is knowing when to let go of your former identity and wear the right hat at the right time.” (14:24) “Make better things for people that will improve their life and their outcomes and the business value will follow if you’ve properly aligned those two things together.” (17:21) “The big message here is this: there is always a design and experience, whether it is an API, or a platform, a dashboard, a full application, etc. Since there are no null design choices, how much are you going to intentionally shape that UX, or just pray that it comes out good on the other end? Prayer is not really a reliable strategy. If you want to routinely do this work right, you need to put intention behind it.” (22:33) “Relationship building is a must, and this is where applying user experience research can be very useful—not just for users, but also with stakeholders. It’s learning how to ask really good questions and learning the feelings, emotions, and reasons why people ask your team to build the thing