Building A Business Powered By Machine Learning At Assembly AI
AI Engineering Podcast - Een podcast door Tobias Macey
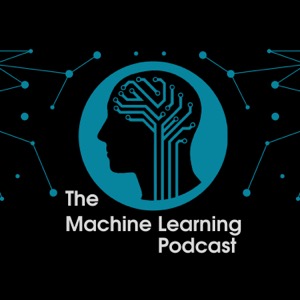
Categorieën:
SummaryThe increasing sophistication of machine learning has enabled dramatic transformations of businesses and introduced new product categories. At Assembly AI they are offering advanced speech recognition and natural language models as an API service. In this episode founder Dylan Fox discusses the unique challenges of building a business with machine learning as the core product.AnnouncementsHello and welcome to the Machine Learning Podcast, the podcast about machine learning and how to bring it from idea to delivery.Predibase is a low-code ML platform without low-code limits. Built on top of our open source foundations of Ludwig and Horovod, our platform allows you to train state-of-the-art ML and deep learning models on your datasets at scale. Our platform works on text, images, tabular, audio and multi-modal data using our novel compositional model architecture. We allow users to operationalize models on top of the modern data stack, through REST and PQL – an extension of SQL that puts predictive power in the hands of data practitioners. Go to themachinelearningpodcast.com/predibase today to learn more and try it out!Your host is Tobias Macey and today I’m interviewing Dylan Fox about building and growing a business with ML as its core offeringInterviewIntroductionHow did you get involved in machine learning?Can you describe what Assembly is and the story behind it? For anyone who isn’t familiar with your platform, can you describe the role that ML/AI plays in your product?What was your process for going from idea to prototype for an AI powered business? Can you offer parallels between your own experience and that of your peers who are building businesses oriented more toward pure software applications?How are you structuring your teams?On the path to your current scale and capabilities how have you managed scoping of your model capabilities and operational scale to avoid getting bogged down or burnt out?How do you think about scoping of model functionality to balance composability and system complexity?What is your process for identifying and understanding which problems are suited to ML and when to rely on pure software?You are constantly iterating on model performance and introducing new capabilities. How do you manage prototyping and experimentation cycles? What are the metrics that you track to identify whether and when to move from an experimental to an operational state with a model?What is your process for understanding what’s possible and what can feasibly operate at scale?Can you describe your overall operational patterns delivery process for ML?What are some of the most useful investments in tooling that you have made to manage development experience for your teams?Once you have a model in operation, how do you manage performance tuning? (from both a model and an operational scalability perspective)What are the most interesting, innovative, or unexpected aspects of ML development and maintenance that you have encountered while building and growing the Assembly platform?What are the most interesting, unexpected, or challenging lessons that you have learned while working on Assembly?When is ML the wrong choice?What do you have planned for the future of Assembly?Contact Info@YouveGotFox on TwitterLinkedInParting QuestionFrom your perspective, what is the biggest barrier to adoption of machine learning today?Closing AnnouncementsThank you for listening! Don’t forget to check out our other shows. The Data Engineering Podcast covers the latest on modern data management. Podcast.__init__ covers the Python language, its community, and the innovative ways it is being used.Visit the site to subscribe to the show, sign up for the mailing list, and read the show notes.If you’ve learned something or tried out a project from the show then tell us about it! Email [email protected]) with your story.To help other people find the show please leave a review on iTunes and tell your friends and co-workersLinksAssembly AIPodcast.__init__ EpisodeLearn Python the Hard WayNLTKNLP == Natural Language ProcessingNLU == Natural Language UnderstandingSpeech RecognitionTensorflowr/machinelearningSciPyPyTorchJaxHuggingFaceRNN == Recurrent Neural NetworkCNN == Convolutional Neural NetworkLSTM == Long Short Term MemoryHidden Markov ModelsBaidu DeepSpeechCTC (Connectionist Temporal Classification) Loss ModelTwilioGrid SearchK80 GPUA100 GPUTPU == Tensor Processing UnitFoundation ModelsBLOOM Language ModelDALL-E 2The intro and outro music is from Hitman’s Lovesong feat. Paola Graziano by The Freak Fandango Orchestra/CC BY-SA 3.0